When it comes to security breaches, it’s not a matter of “if,” but “when.” The time has come for security practitioners to start considering not only how to protect the systems holding the data, but also how to make datasets themselves less toxic and privacy-invasive when breached.
That is where privacy engineering comes in.
“Privacy Engineering is the translation of privacy policies and cultural preferences into testable tools, algorithms, and models that can be utilized to build privacy-sensitive systems,” explained Dr. Daniel Aranki, an I School lecturer and postdoctoral scholar and CLTC grantee.
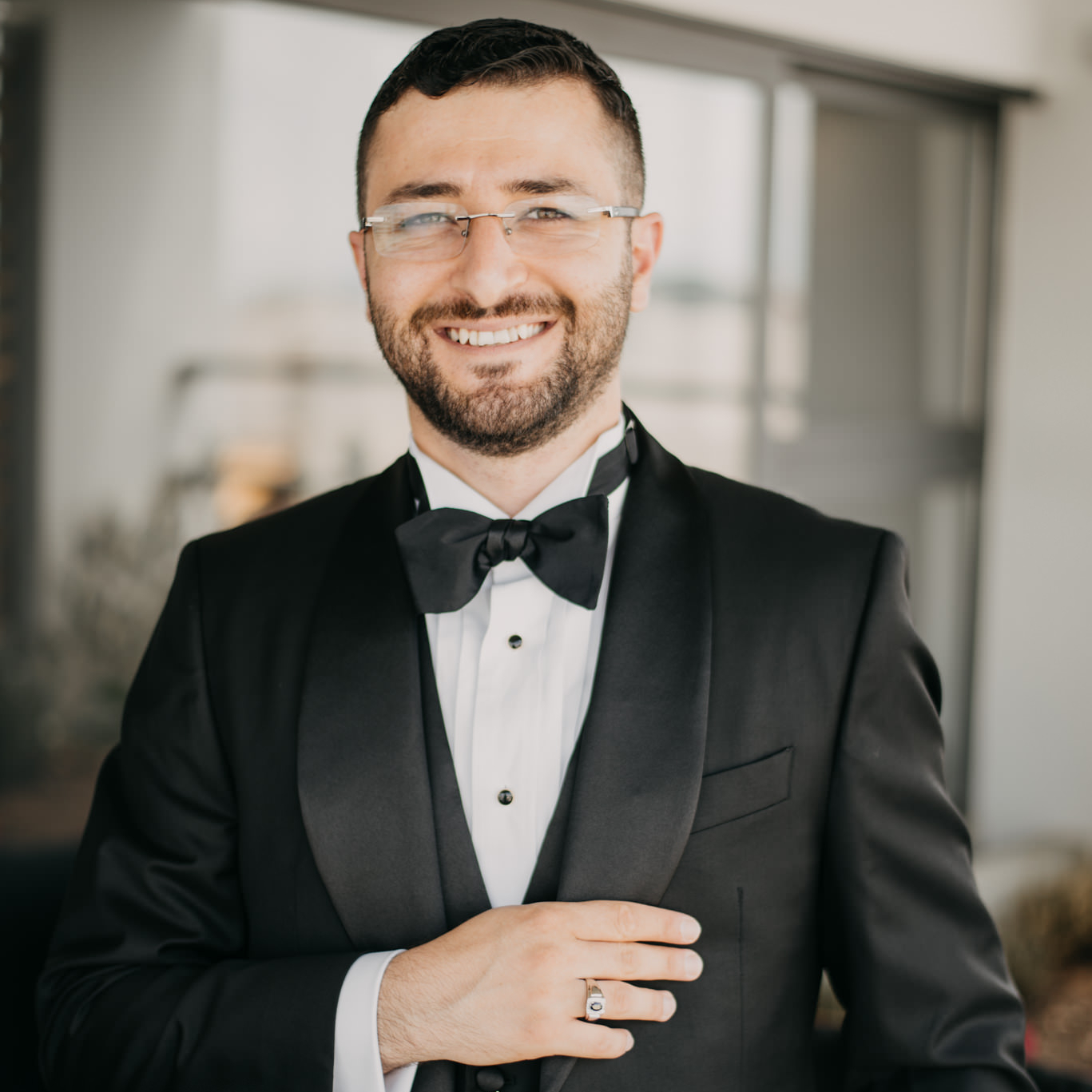
Dr. Aranki has been teaching Privacy Engineering to Master of Information and Cybersecurity (MICS) students since the spring of 2019, and this semester Introduction to Privacy Engineering is being offered for the first time to on-campus graduate and undergraduate students as INFO 290.
“Privacy Engineering involves a set of skills which enable cybersecurity professionals to not only design privacy-aware systems,” said Dr. Aranki, “but also design security-critical systems in a manner that assumes breach and minimizes impact when such a breach occurs.”
This past summer, MICS students armed with final projects from the course have hit the conference circuit to share their research.
At DataCon LA on August 17, MICS students Ken Chang and Serena Villalobos presented their research demonstrating how current HITRUST Common Security Framework, a widely-used security framework intended to protect confidential health information, and NIST Special Publication 800-53, which provides a catalog of security and privacy controls for all U.S. federal information systems, are insufficient to protect the anonymity of patient records in the Surveillance, Epidemiology, and End Results (SEER) database of the National Cancer Institute.

This research project was done in collaboration with Heather McPherson and Matthew Holmes. “The students applied models and principles taught in class, such as k-anonymity, to not only show the insufficient protections to anonymity in the SEER database but to also demonstrate how acceptable levels of privacy can be achieved,” Dr. Aranki explained.
k-Anonymity is a property of certain data sets, in which data have been cleaned to protect anonymity.
At the USENIX Security ’19 Lightning Talks on August 14, Jacob (Jake) Lin revealed the very personal inferences that can be drawn from public Venmo data. Pascal Issa and Daniel Bozinov also contributed to this project. “In class, we discuss the inference threat at length and the students did a great job to demonstrate this threat on a real-world dataset,” Dr. Aranki shared. “A future direction for this project would be to apply privacy engineering principles to protect against these inferences.”
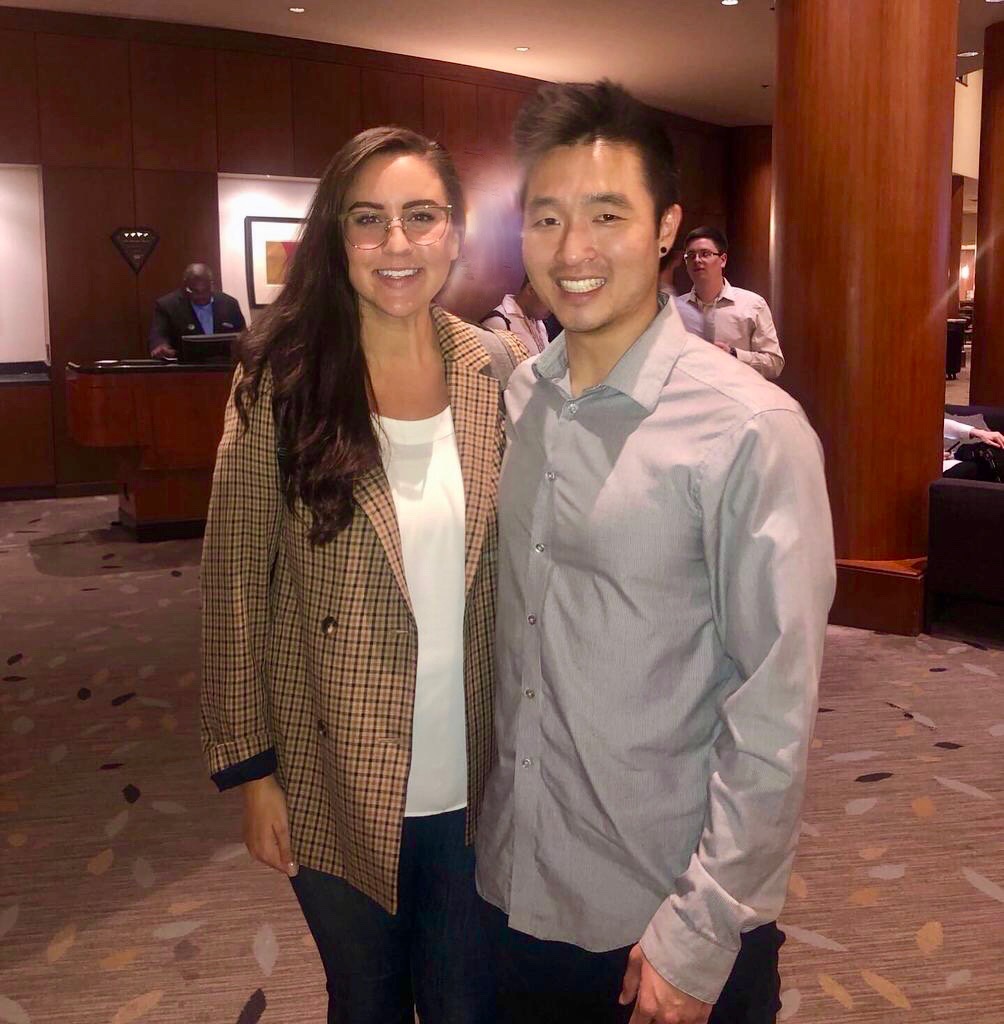
Also at the USENIX Security ’19 Lightning Talks, Stephanie Perkins and Jacob Bolotin shared how data anonymization techniques could encourage organizations with data relevant to sex-trafficking investigations to publish their datasets. Nugzar Nebieridze was also a member of the project team. “This is an innovative use of privacy protections, including k-anonymity and l-diversity, to minimize the potential legal liability of organizations when they share internal datasets that are relevant to fighting human- and sex-trafficking,” Dr. Aranki said.
l-Diversity is an extension of k-anonymity to further maintain the diversity of sensitive data fields.
“Our objectives with this course revolve around introducing students to the field of Privacy Engineering and bringing them to a level where they are able to stay up-to-date with the state-of-the-art in the field,” explained Dr. Aranki. “We are delighted that these objectives were exceeded. Every semester so far, Privacy Engineering students have produced novel research in the field and presented their work at professional conferences.”