By UC Berkeley Division of Data Science and Information
UC Berkeley Assistant Professor Zachary Pardos and his team have developed a machine learning approach that promises to help more community college students position themselves to transfer and succeed at four-year colleges and universities.
Along the way, they’ve discovered that considering course enrollment patterns — or the classes that students take before, along with, and after a particular course — can help provide a more complete picture of what courses should “count” when students transfer.
Roughly 80% of community college students aim to continue their education at four-year institutions, but the vast majority never make the transfer. Contributing to the problem are the complexities of “articulation,” or determining which course at one institution will count for credit at another. This entails assessing the similarity of thousands, or potentially even millions, of pairs of courses, an endeavor that’s impossible to comprehensively achieve and keep current across all institutions manually.
So Pardos, an Assistant Professor at Berkeley’s Graduate School of Education and School of Information, has developed a machine learning approach that automates these initial assessments and develops suggestions for pairings. The approach also identifies potential “unpairings” of existing matches that may be out of date or misaligned in other ways. These suggestions can then be presented to students or administrators, enabling both to consider a much wider range of potential articulations than they would otherwise have the time and resources to examine.
Using a set of approved pairings, Pardos and his team investigated different techniques for automating course comparisons. In addition to using natural language processing to compare descriptions in course catalogs, they analyzed millions of historic course enrollments to get a more comprehensive picture. “Catalog descriptions are an expected source of course similarity across institutions,” Pardos said. “Interestingly, we found that the contexts in which a course is taken — for instance, the other courses taken in the same or adjacent terms — conveys almost as much information about the course.”
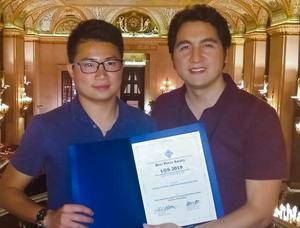
Ultimately, they found that it was most effective to use both sources of information. Since catalog descriptions can be brief, generic, and out of date, also looking at the classes that students take before and after a given course can help develop a better understanding of which courses at community colleges best map to those at four-year institutions. This method opens new possibilities for course pairings that might not otherwise surface, including those between different disciplines or institutions that may not have been linked previously.
Pardos hopes to expand the use of this method at Berkeley and beyond. His paper describing the approach, Data- Assistive Course-to-Course Articulation Using Machine Translation, co-authored by visiting undergraduate student Haocheng Zhao at UC Berkeley and doctoral student Hung Chau at the University of Pittsburgh, was named best paper at the 2019 Learning @ Scale conference. After presenting the paper, Pardos received an award to implement this data-assistive articulation work at two of the 19 City University of New York (CUNY) system institutions.
Originally published as Using Machine Learning to Broaden Pathways from Community College by the UC Berkeley Division of Data Science and Information on September 30, 2019.