Ph.D. student Lucy Li Awarded Best Paper by the American Educational Research Association
Because textbooks are the most widely used instructional materials in the world, their content is especially important. They have the potential to impact students’ cultural and societal beliefs about people of different races, backgrounds, and gender, but most content analysis of texts relies on hand-coding, which is both labor-intensive and hard to scale. School of Information Ph.D. student Lucy Li and collaborators Dorottya Demszky, Patricia Bromley, and Dan Jurafsky set out to apply natural language processing (NLP) to textbook content to answer questions that textbook researchers in education care about, and their findings were awarded Best Paper by the American Educational Research Association.
The paper, “Content Analysis of Textbooks via Natural Language Processing: Findings on Gender, Race and Ethnicity in Texas U.S. History Textbooks” was presented at the AERA Conference on Educational Data Science at Stanford University in September 2020. The authors asked three questions that are of particular interest to textbook education researchers:
- How much are different groups of people mentioned?
- How are different groups and individuals described?
- What Are Prominent Topics and How Are they Related to Groups of People?
The results found quantitative and scaleable measurements of textbook content: while 52% of students in Texas are Latinx, there is little mention of them across all textbooks; in fact, only 0.2% of common nouns refer to Latinx people. White men who are political leaders are the most frequently named people across textbooks, and words most often used to describe them are words such as powerful, young, and military.
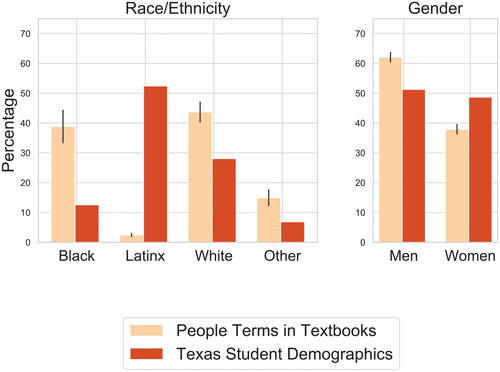
Common descriptors for Blacks are words such as escaped, owned, freed. Analysis of verbs show Black people were most often depicted performing actions associated with low agency and power, and that women are most often mentioned in the context of marriage and home, with married, young, and unmarried being the most frequently used descriptors of women.
Natural language processing uses algorithms to analyze, understand, and attain meaning from human language. The researchers examined 15 of the most widely used textbooks in Texas using NLP to demonstrate methods for quantifying the content of textbooks that connect to the social sciences, policy, and practical aims of educational research.
The researchers chose Texas as their focus because its data was readily available online, and because the state has the second-largest student population in the U.S, with 5.4 million students enrolled in its public schools in 2017. Because of Texas’ size and subsequent purchasing power, the state’s content preferences have a significant impact on textbook content across the country. The team’s research sought to use NLP to examine depictions of historically marginalized groups such as women, Black and Latinx people.
Li and her collaborators see textbooks as a fecund environment for the application of NLP methods. Computational tools will enable the capacity for larger and more robust social science studies and will allow for a deeper understanding of links between texts and social influences.
The research is pioneering: most NLP methods are optimized for other domains. By using textbooks as a source, Li and her collaborators have opened a door for education researchers. “Textbooks and other educational materials are new datasets that offer new questions to think about,” Li said.
The interdisciplinary team included not only computer scientists, linguists, and NLP researchers, but a Stanford education professor. “It was exciting,” Li said, “to collaborate on this project with someone who wasn’t coming to this from a computer science or linguistics background to see what (research questions) they spend their time thinking about.”
“This work has been presented at several venues over the course of its development,” said Li’s advisor, School of Information Professor David Bamman, “and has been extraordinarily well received at each one. At the 2019 Text as Data conference, it was one of the most buzzworthy of the circa 20 oral presentations given. I have every expectation that this work will show up on course syllabi exemplifying the best use of NLP in computational social science.”